Table Of Content

Of note, the block effect is typically considered as a random effect. Finally, if you expect the 'treatment effect' to differ from block to block, then interactions should be considered. A similarapproach can also be used in untargeted settings, both with and withoutlabels,12,23,24 where onesample is used as a standard throughout the experiment. Having a commonreference makes samples more easily comparable across the differentsettings (e.g., batches, days of analysis, and instruments) by providinga common baseline. However, this only works for processing steps thatthe reference sample shares with the other samples in the relevantbatch, and poses challenges in terms of missing value and dynamicrange that are beyond the scope of this article. Most of thevariables inspected so far have divided the samples into nonoverlappingcategories, this will however not always be the case.
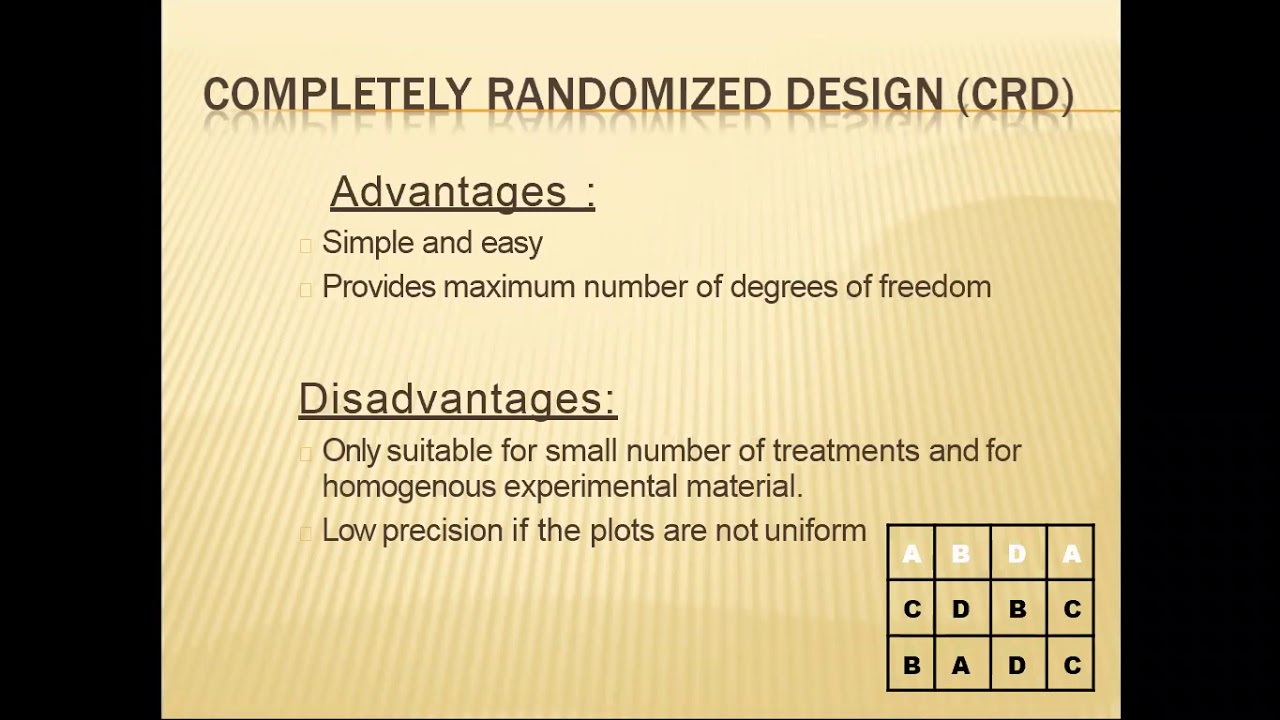
Model
These designs were especially useful in applications of the technique of analysis of variance (ANOVA). Here, the condition that any x in X is contained in r blocks is redundant, as shown below. In statistics, the concept of a block design may be extended to non-binary block designs, in which blocks may contain multiple copies of an element (see blocking (statistics)). There, a design in which each element occurs the same total number of times is called equireplicate, which implies a regular design only when the design is also binary. The incidence matrix of a non-binary design lists the number of times each element is repeated in each block.
Identify nuisance variables
Block randomization is an approach thatcan prevent severe imbalances in sample allocation with respect toboth known and unknown confounders. This feature provides the readerwith an introduction to blocking and randomization, and insights intohow to effectively organize samples during experimental design, withspecial considerations with respect to proteomics. The mathematical subject of block designs originated in the statistical framework of design of experiments.
Redesigning the Grid: Barcelona's Experiment with Superblocks - Urban Land Magazine - Urban Land
Redesigning the Grid: Barcelona's Experiment with Superblocks - Urban Land Magazine.
Posted: Mon, 18 Dec 2017 08:00:00 GMT [source]
3 - The Latin Square Design
The third case, where the replicates are different factories, can also provide a comparison of the factories. The fact that you are replicating Latin Squares does allow you to estimate some interactions that you can't estimate from a single Latin Square. If we added a treatment by factory interaction term, for instance, this would be a meaningful term in the model, and would inform the researcher whether the same protocol is best (or not) for all the factories.
Block Randomization
So, consider we had a plot of land, we might have blocked it in columns and rows, i.e. each row is a level of the row factor, and each column is a level of the column factor. We can remove the variation from our measured response in both directions if we consider both rows and columns as factors in our design. The original use of the term block for removing a source of variation comes from agriculture. If the section of land contains a large number of plots, they will tend to be very variable - heterogeneous.
Sample Randomization
Finally, we walk through the steps that you need to take in order to implement blocking in your own experimental design. This activity walks students through three different experimental designs, in increasing complexity. Now let us introduce a machine drift (Figure Figure11C,G) that causesthe mass spectrometer todetect slightly less of the protein over time. For the ordered allocation,the observed protein abundances show almost no difference betweenthe two group means (Figure Figure11D). Conversely, the difference in group means for the randomizedallocation is nearly the same as the “true” difference(Figure Figure11H), and onlyhas added variance caused by the machine drift (Figure Figure22).
Example Problem on Randomized Complete Block Design
The RCBD utilizes an additive model – one in which there is no interaction between treatments and blocks. The error term in a randomized complete block model reflects how the treatment effect varies from one block to another. In our previous diet pills example, a blocking factor could be the sex of a patient. And within each of the two blocks, we can randomly assign the patients to either the diet pill (treatment) or placebo pill (control). By blocking on sex, this source of variability is controlled, therefore, leading to greater interpretation of how the diet pills affect weight loss.
In that situation, randomized block design can decreases the statistical power and thus be worse than a simple single-factor between-subjects randomized design. Again, your best bet on finding an optimal number of blocks is from theoretical and/or empirical evidences. Randomized block design still uses ANOVA analysis, called randomized block ANOVA.
To achieve replicates, this design could be replicated several times. Here is a plot of the least squares means for Yield with the missing data, not very different. There are 23 degrees of freedom total here so this is based on the full set of 24 observations. In that sense, Latin Square designs are useful building blocksof more complex designs, see for example Kuehl (2000). Why is it important to make sure that the number of soccer players running on turf fields and grass fields is similar across different treatment groups? In other words, when the error term is inflated, the percentage of variability explained by the statistical model diminishes.
Subjects are randomly assigned to a block and the order ofthe subjects within each block is randomized. Ok, with this scenario in mind, let's consider three cases that are relevant and each case requires a different model to analyze. The cases are determined by whether or not the blocking factors are the same or different across the replicated squares. The treatments are going to be the same but the question is whether the levels of the blocking factors remain the same. Whenever, you have more than one blocking factor a Latin square design will allow you to remove the variation for these two sources from the error variation.
Ideally, experiments should be run by using completely randomized experimental units. However, often, there is not enough experimental units from one homogenous sample. For example, if there are not enough raw materials to produce all the experimental units for all replications, blocking is utilized to control the nuisance effects of the experimental units coming from, possibly non homogenous batches. Different batches do not necessarily mean non-homogeneity all the time. However, keeping track of the batch numbers as blocks (the statistical term) would provide an opportunity, if in case there is non-homogeneity from batch to batch. Therefore, a block is defined by a homogenous large unit, including, raw materials, areas, places, plants, animals, humans, etc. where samples or experimental units drawn are considered identical twins, but independent.
A randomized block design with the following layout was used to compare 4 varieties of rice in 5 blocks. The first step of implementing blocking is deciding what variables you need to balance across your treatment groups. Here are some examples of what your blocking factor might look like. So what types of variables might you need to balance across your treatment groups?
It would reduce the overall effect of that treatment, and the estimated treatment mean would be biased. After calculating x, you could substitute the estimated data point and repeat your analysis. So you can analyze the resulting data, but now should reduce your error degrees of freedom by one. In any event, these are all approximate methods, i.e., using the best fitting or imputed point.
No comments:
Post a Comment